Artificial Intelligence (AI) has rapidly evolved from a futuristic concept to a cornerstone of modern technology. From natural language processing and computer vision to autonomous vehicles and virtual assistants, AI is reshaping industries and transforming the way we interact with technology. As we continue to push the boundaries of what AI can achieve, it’s clear that networking infrastructure plays a pivotal role in harnessing its full potential.
The AI Revolution: What’s Driving the Change?
Recent advances in AI, especially in generative models that create text and images, have sparked widespread fascination. Tools like ChatGPT and Google LaMDA are not just answering questions but also crafting essays, coding applications, and even composing music. This surge in AI capabilities is not just a technological leap but a profound shift in how various job functions are executed.
Developing an AI model is a complex process involving three key phases:
- Data Preparation: Gathering and curating datasets to feed into the AI model.
- AI Training: Teaching the model by exposing it to vast amounts of data to recognize patterns and relationships.
- AI Inference: Applying the trained model to real-world scenarios, making predictions or decisions based on new data.
This training process is iterative, refining the model’s parameters and improving its accuracy over time. However, as AI models become more advanced, especially in deep learning, the demands on compute resources increase significantly. Training a cutting-edge model can be extremely costly, with expenses often exceeding $400,000 per GPU-powered AI training server.
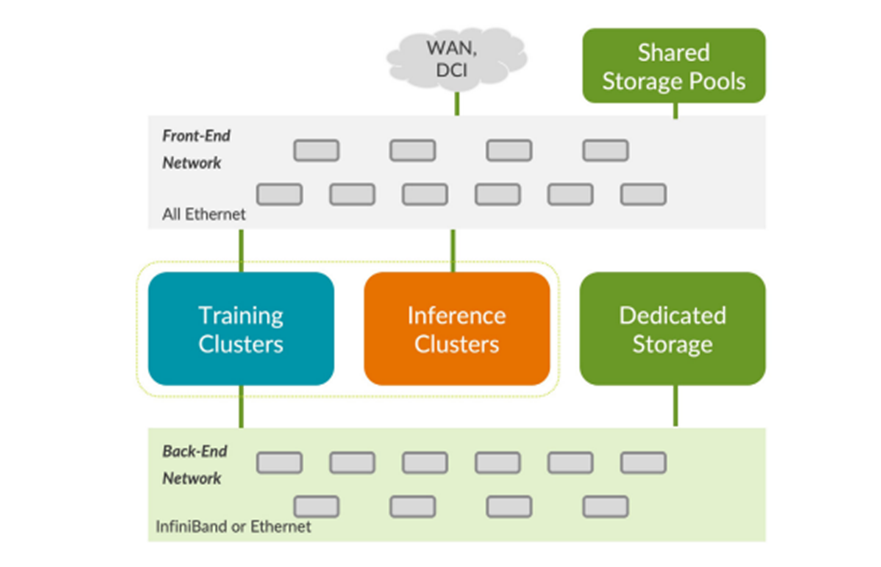
The Network’s Role in AI Training: The Bottleneck Challenge
AI training involves substantial data transfer between GPUs, necessitating efficient networking to avoid bottlenecks. The performance of AI training is deeply intertwined with network efficiency. Traditional data centre networks, designed for cloud computing, struggle to handle the specific needs of AI workloads. High-bandwidth, low-latency network fabrics are required to support the massive data flow and avoid congestion.
Key Challenges in AI Networking:
- Leaf-to-Spine Uplink Congestion: GPUs can consume network capacity rapidly, leading to potential congestion if load balancing is uneven.
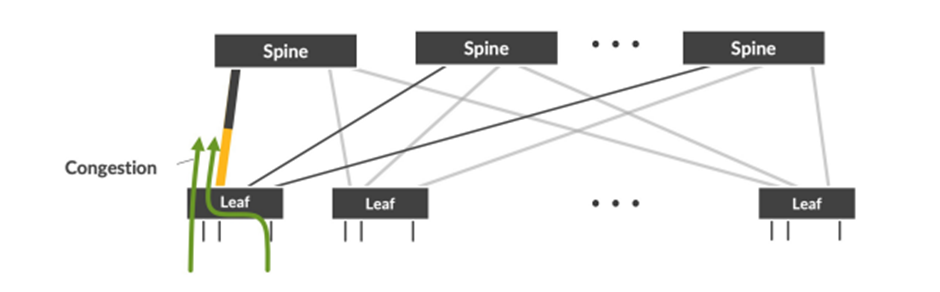
- Spine-to-Leaf Downlink Congestion: Traffic directed to a single leaf switch can oversubscribe the interface, causing congestion and reduced efficiency.
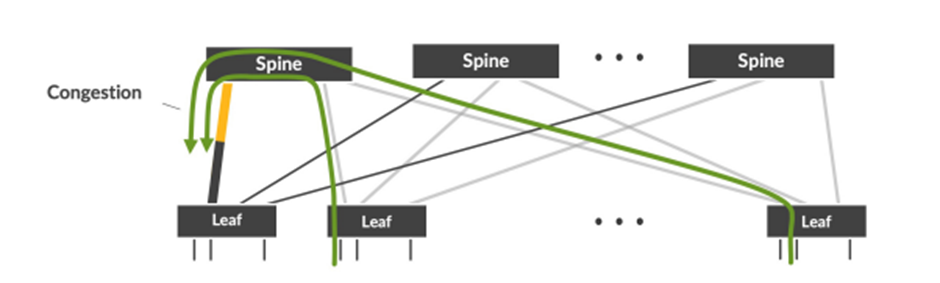
To address these issues, effective load balancing, congestion management, and high-capacity networking are essential. As AI models scale, network designs must evolve to support high bandwidth and low latency while avoiding packet drops and retransmissions.
Ethernet vs. InfiniBand: The Networking Showdown
Historically, InfiniBand was favoured for its high-speed, low-latency communication, but Ethernet is rapidly gaining ground as the preferred technology for AI networking. Ethernet’s open standard and broad adoption offer several advantages:
- Cost-Effectiveness: Ethernet solutions are generally less expensive than proprietary InfiniBand technologies.
- Flexibility: Ethernet supports a wider range of vendors and tools, making it easier to integrate and manage.
- Scalability: Advancements in Ethernet technology, such as RoCE (RDMA over Converged Ethernet), enable high-speed data transfer that meets the needs of modern AI applications.
Ethernet’s ability to evolve and support high-capacity, low-latency networking makes it an ideal choice for AI infrastructure. Its open nature fosters innovation and reduces costs, making it a practical solution for both private and public AI deployments.
Designing the Ideal AI Network Fabric
To optimize AI training efficiency, a robust network design is crucial. Juniper Networks advocates for a high-capacity, non-blocking Ethernet fabric with:
- High-Capacity Switches: For instance, Juniper’s PTX10000 routers and QFX5200 switches provide the necessary performance and scalability.
- Efficient Load Balancing: Advanced algorithms and technologies like Explicit Congestion Notification (ECN) and Priority-Based Flow Control (PFC) help manage traffic and reduce congestion.
- Adaptive Load Balancing: Monitoring and adjusting traffic flow dynamically to ensure optimal network utilization.
These design principles help ensure that AI infrastructure can handle the intense demands of training and inference, maximizing GPU efficiency and minimizing job completion time.
Looking Ahead: The Future of AI and Networking
As AI technology continues to advance, the role of networking will become even more critical. Efficient, high-capacity, and flexible networking solutions will be vital to support the next generation of AI applications. Juniper Networks is committed to delivering cutting-edge AI infrastructure solutions built on open, standards-based Ethernet technologies. By focusing on scalability, cost-effectiveness, and operational simplicity, Juniper aims to drive the future of AI networking.
In summary, while AI is making tremendous strides, the underlying infrastructure that supports it, particularly networking, is key to unlocking its full potential. As we continue to explore the boundaries of AI, ensuring that our network designs can keep up with its demands will be crucial for future success.